Recently, Deep Learning has produced rapid advances in the performance of medical image analysis challenging physicians in their traditional field. In pathology in particular, automated procedures can help to reduce the pathologists' workload and increase the accuracy and precision of pathology assessments, which are often considered subjective and with less than optimal reproducibility. In addition, Deep Learning and Computer Vision demonstrate the ability/potential to extract more clinically relevant information from whole slide images, compared to what is possible in current routine clinical practise by human assessors. Nevertheless, considerable development and validation work lies ahead before AI-based methods are ready for integration into pathology departments.
The size of whole-slide image data, the weak annotation of specimens, the variability in slide preparation due to different procedures (e.g., staining, digitization) make the learning environment a major challenge, even for state-of-the-art algorithms, requiring further contributions from the Computer Vision community. Moreover, the need for transparent decision making further increases the challenge.
The workshop on Computational Challenges in Digital Pathology (CDpath) at ICCV 2021 aims to foster discussion and presentation of ideas to tackle these challenges and identify research opportunities in the context of Computational Pathology.
Opening session
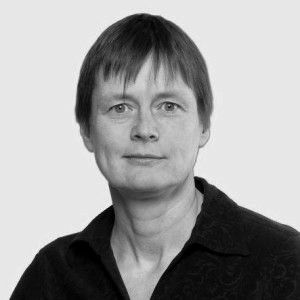
Keynote talk: Prof. Anne Martel
Applying AI to whole slide pathology images for the assessment of residual breast cancer
(30 min presentation + 10 min Q&A)
Abstract: Neoadjuvant chemotherapy (NAC) is the systemic treatment of breast cancer patients prior to surgery.
The response to NAC can guide treatment decisions post-operatively and it is assessed by examining the excised tissue from the
primary tumor site and resected lymph nodes. The residual cancer burden (RCB) index, proposed by Symmans et al, incorporates measurements
of the primary tumour with an assessment of metastatic spread in the lymph nodes and this measure is predictive of long-term survival
after NAC. In practice, however, the assessment of RCB is too time consuming and most pathologists simply report whether there is a complete
response or residual tumour. We have been working closely with breast pathologists to develop AI models that can carry out the RCB
assessment automatically. In this talk I will outline some of the challenges we have encountered when working with WSIs which include working
with limited data sets, and with sparse or incomplete annotations. I will also present some of approaches we have taken to overcome these challenges.
About Anne Martel: Dr. Anne Martel is a Professor in Medical Biophysics at the University of Toronto, a Senior Scientist at Sunnybrook
Research Institute, and a Vector Faculty Affiliate. Her research program, funded by CIHR, NSERC, NIH and the Canadian Cancer Society, is focused
on medical image and digital pathology analysis, particularly on applications of machine learning for segmentation, diagnosis, and prediction/prognosis.
In 2006 she co-founded Pathcore, a software company developing complete workflow solutions for digital pathology. Dr. Martel is an active member of
the medical image analysis community and is a fellow of the MICCAI Society which represents engineers and computer scientists working in this field.
She has served as board member of MICCAI and as an Associate Editor of IEEE Transactions on Medical Imaging.
Oral session I: full presentations
(10 min presentations + 5 min Q&A)
"Guided Representation Learning for the Classification of Hematopoietic Cells"
by Philipp Gräbel, Martina Crysandt, Barbara M. Klinkhammer, Peter Boor, Tim H. Brümmendorf and Dorit Merhof
"H&E-adversarial network: a convolutional neural network to learn stain-invariant features through Hematoxylin & Eosin regression"
by Niccolò Marini, Manfredo Atzori, Sebastian Otálora, Stephane Marchand-Maillet and Henning Müller
"Self-Supervised Representation Learning using Visual Field Expansion on Digital Pathology"
by Joseph Boyd, Mykola Liashuha, Eric Deutsch, Nikos Paragios, Stergios Christodoulidis and Maria Vakalopoulou
"ALBRT: Cellular Composition Prediction in Routine Histology Images"
by Muhammad Dawood, Kim Branson, Nasir Rajpoot and Fayyaz Minhas
"Robust Interactive Semantic Segmentation of Pathology Images with Minimal User Input"
by Mostafa Jahanifar, Neda Z. Tajeddin, Navid A. Koohbanani and Nasir Rajpoot
Coffee Break
Oral session II: pitch presentations
(5 min presentations)
"Simultaneous Nuclear Instance and Layer Segmentation in Oral Epithelial Dysplasia"
by Adam J. Shephard, Simon D. Graham, Saad Bashir, Mostafa Jahanifar, Hanya Mahmood, Ali Khurram and Nasir Rajpoot
"Improving Self-supervised Learning with Hardness-aware Dynamic Curriculum Learning: An Application to Digital Pathology"
by Chetan L. Srinidhi and Anne Martel
"Probeable DARTS with Application to Computational Pathology"
by Sheyang Tang, Mahdi S. Hosseini, Lina Chen, Sonal Varma, Corwyn Rowsell, Savvas Damaskinos, Konstantinos N. Plataniotis and Zhou Wang
"Iterative Cross-Scanner Registration for Whole Slide Images"
by Luisa Theelke, Frauke Wilm, Christian Marzahl, Christof Bertram , Robert Klopfleisch, Andreas K. Maier, Marc Aubreville and Katharina Breininger
"Joint Semi-supervised and Active Learning for Segmentation of Gigapixel Pathology Images with Cost-Effective Labeling"
by Zhengfeng Lai, Chao Wang, Luca C. Oliveira, Brittany N. Dugger, Sen-Ching S. Cheung and Chen-Nee Chuah
"An investigation of attention mechanisms in histopathology whole-slide-image analysis for regression"
by Philippe Weitz, Yinxi Wang, Johan Hartman and Mattias Rantalainen
"Multi-Prototype Few-shot Learning in Histopathology"
by Jessica Deuschel, Daniel Firmbach, Carol Geppert, Markus Eckstein, Arndt Hartmann, Volker Bruns, Petr Kuritcyn, Jakob Dexl, David Hartmann, Dominik Perrin,
Thomas Wittenberg and Michaela Benz
"A Pathology Deep Learning System Capable of Triage of Melanoma Specimens Utilizing Dermatopathologist Consensus as Ground Truth"
by Sivaramakrishnan Sankarapandian, Saul Kohn, Vaughn Spurrier, Sean Grullon, Rajath E. Soans, Kameswari D. Ayyagari, Ramachandra V. Chamarthi, Kiran Motaparthi, Jason B. Lee, Wonwoo
Shon, Michael Bonham and Julianna D. Ianni1
"A QuadTree Image Representation for Computational Pathology"
by Robert Jewsbury, Abhir Bhalerao and Nasir Rajpoot
"Deep Ordinal Focus Assessment for Whole Slide Images"
by Tomé Albuquerque, Ana Moreira and Jaime S. Cardoso
"Lizard: A Large-Scale Dataset for Colonic Nuclear Instance Segmentation and Classification"
by Simon D. Graham, Mostafa Jahanifar, Ayesha Azam, Mohammed Nimir, Yee-Wah Tsang, Katherine Dodd, Emily Hero, Harvir Sahota, Atisha Tank, Ksenija Benes, Noorul Wahab,
Fayyaz Minhas, Shan Raza, Hesham El Daly, Kishore Gopalakrishnan, David Snead and Nasir Rajpoot
"Real-Time Cell Counting in Unlabeled Microscopy Images"
by Yuang Zhu, Zhao Chen, Yuxin Zheng, Qinghua Zhang and Xuan Wang
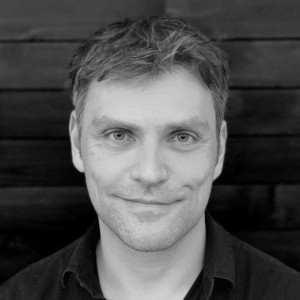
Keynote talk: Dr. Martin Stumpe
The Role of Digital Pathology in a Multimodal AI Precision Medicine Environment
(30 min presentation + 10 min Q&A)
Abstract: Precision medicine is increasingly leveraging data from multiple modalities for creating more comprehensive
insights into the diagnostic state of a patient, and more accurate predictions of their expected health trajectory. While genomic data from DNA- and
RNA-sequencing yields salient information about a patient’s molecular priors, and clinical data about their historic disease context, imaging has the
unique ability to yield critical information about the spatial disease context, e.g. of the tumor microenvironment in oncology. This talk addresses how
these different modalities can be used in a multimodal AI precision medicine environment, with example applications ranging across biomarker identification,
clinical trial enrollment, risk and response predictions, and operational improvements using digital pathology.
About Martin Stumpe: Dr. Martin Stumpe serves as the Chief of AI at Tempus. Prior to joining Tempus, he founded and led the Cancer Pathology project
at Google, which uses artificial intelligence to increase the accuracy in image-based cancer detection and grading. His team also succeeded in integrating
these diagnostics into optical microscopy for real-time augmented intelligence. Earlier in his career, Dr. Stumpe was part of the Kepler team at NASA Ames Research
Center in Mountain View, California, where he was responsible for the development of algorithms to detect extrasolar planets from stellar light curves. Before that,
he was a postdoctoral researcher at Stanford University in the lab of Vijay Pande, focusing on molecular dynamics simulations to study chaperone-induced protein
folding. During this time, he also developed and brought to market a particle tracking software which is used primarily in ethological studies. Dr. Stumpe holds a
PhD in Computational and Theoretical Physics from the Max-Planck-Institute for Biophysical Chemistry in Goettingen, Germany.
Closing session
* All times are EDT - Eastern Daylight Time *
The CDpath workshop aims at providing a platform for scientific discussion on computational pathology, introducing the challenges of the field to the Computer Vision and Artificial Intelligence community.
The CDpath workshop welcomes works that focus on the computational pathology challenges, such as:
Semi/unsupervised learning methodologies in computational pathology;
Detection, classification and segmentation of tissue structures;
Disease diagnosis, grading and prognosis;
Treatment response prediction;
Staining quality assessment and standardization;
Detection of tissue biomarkers with predictive/prognostic value;
Whole-slide image registration;
Explainable AI for computational pathology;
Scalable techniques for whole-slide image processing;
Applications for computational pathology in the clinic.
Authors should prepare a manuscript of no more than 8 pages, including images and tables (with possible extra pages containing only cited references).
The manuscript submitted to the workshop should be formatted according to the ICCV 2021 and anonymised.
Papers that are not properly anonymised, or do not use the template, or have more than 8 pages (excluding references) will be desk-rejected.
Authors can also submit supplementary material and code, provided it is in pdf or zip format (only), anonymised and properly referenced in the paper.
Please refer to the ICCV 2021 submission guidelines
for more details (sections 6 to 8).
All submissions will be evaluated by 3 reviewers, in a double-blind reviewing process. Authors/reviewers will be asked to disclose any potential conflicts of interest,
such as collaborations in the last 3 years. The selection of the papers will be based on their relevance for the computational pathology topic, technical
and experimental quality, significance of results, and clear presentation.
The workshop accepted papers will be published in IEEE Xplore in conjunction with ICCV 2021 proceedings.
Submission deadline: July 25, 2021* July 28, 2021*
Author notification: August 12, 2021*
Camera ready deadline: August 16, 2021*
CDpath workshop: October 11, 2021
Aïcha Bentaieb,
Senior Machine Learning Scientist at Tempus Labs Inc., USA
Antonio Foncubierta Rodriguez,
Research Staff Member at IBM, Switzerland
Andreas Holzinger,
Head of HCAI Lab at Med Uni Graz & Visiting Professor at TU Wien/Amii, Austria
Apaar Sadhwani,
Research Scientist at Google Health, USA
April Khademi,
Principal Investigator of IAMLAB & Assistant Professor at Ryerson University, Canada
Azam Hamidinekoo,
Postdoctoral Training Fellow at Institute of Cancer Research, UK
Faisal Mahmood,
Head of Mahmood Lab & Assistant Professor at Harvard Medical School, USA
Geert Litjens,
Assistant Professor at Radboud University Medical Center, The Netherlands
Hamid Tizhoosh,
Head of Kimia Lab & Professor at University of Waterloo, Canada
Heather Couture,
ML Consultant/Researcher/Owner at Pixel Scientia Labs, USA
Henning Müller,
Head of MedGIFT Lab & Professor at HES-SO Valais-Wallis & University of Geneva, Switzerland
Jana Lipkova,
Postdoctoral Researcher at Harvard Medical School, USA
Lee Cooper,
Computational Pathology Director/Associate Professor at Northwestern University, USA
Manfredo Atzori,
Senior Researcher at HES-SO Valais-Wallis and Assistant Professor at UNIPD, Switzerland
Maschenka Balkenhol,
Pathology Resident/Researcher at Radboud University Medical Center, The Netherlands
Matthew Lee,
AI Scientist at Paige.AI, UK
Navid Alemi,
AI Scientist at Paige.AI, UK
Peter Bankhead,
Senior Lecturer in Digital Pathology at the University of Edinburgh, Scotland
Peter Schüffler,
Assistant Professor at TUM & Co-founder/Senior AI Scientist at Paige.AI, Germany
Saad Ullah Akram,
MVision AI Co-Founder/CTO & Visiting Postdoctoral Researcher at Aalto University, Finland
Shan Raza,
Assistant Professor at Warwick University & Researcher at TIA Centre, UK
Yun Liu,
Research Scientist at Google Health, USA