Speaker Details
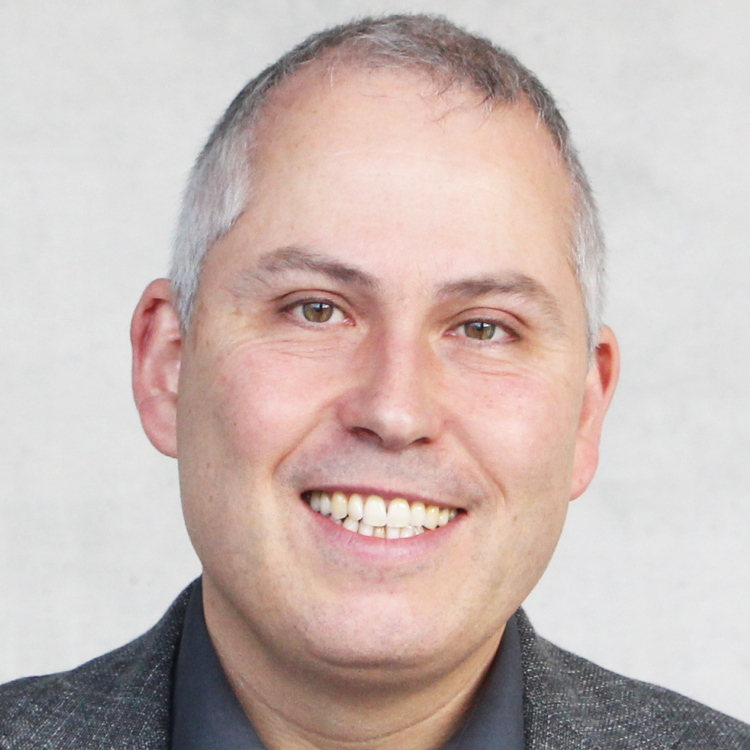
Peter Eisert
IAPR Invited Keynote Speaker
Peter Eisert is Professor for Visual Computing at the Humboldt University Berlin and heading the Vision & Imaging Technologies Department of the Fraunhofer Institute for Telecommunications - Heinrich Hertz Institute Berlin, Germany. He is also a Professor Extraordinaire at the University of Western Cape, South Africa. He received the Dipl.-Ing. degree in Electrical Engineering "with highest honors" from the Technical University of Karlsruhe, Germany, in 1995 and the Dr.-Ing. degree "with highest honors" from the University of Erlangen-Nuremberg, Germany, in 2000.
In 2001, he worked as a postdoctoral fellow at the Stanford University, USA, on 3D image analysis as well as facial animation and computer graphics. In 2002, he joined Fraunhofer HHI, where he is coordinating and initiating numerous national and international 3rd party funded research projects with a total budget of more than 15.6 Million Euros.
He has published more than 150 conference and journal papers and is Associate Editor of the International Journal of Image and Video Processing as well as in the Editorial Board of the Journal of Visual Communication and Image Representation. His research interests include 3D image/video analysis and synthesis, face and body processing, image-based rendering, computer vision, computer graphics in application areas like multimedia, security and medicine.
Keynote Talk:
Explainable AI for Face Morphing Attack Detection
Deep learning has received an enormous interest for many data analysis tasks. In various applications, including biometrics and forensics, such methods outperform classical approaches in terms of accuracy and classification performance. However, deep learning usually provides only black box decisions, which are critical in most security and safety related applications. Here, it is desirable to know, why a neural network has come to a particular decision in order to verify a decision or to modify the system in case of mis-classifications.
In this talk, state of the art methods, like layerwise relevance propagation (LRP), will be presented that enable the explanation and visualization of neural network decisions. This will be illustrated for the particular case of CNN based face morphing attack detection. It is shown that not only plausibility of decisions can be determined but also generality and attack detection performance can be improved, making a system more robust to unknown future threats.